Technology
Innovative Photonic and AI Technology Powering Nutriscope™
Harnessing Photonic Technology for Real-Time Crop Monitoring
Nutriscope™ works by shining light onto a plant and measuring how much is absorbed, reflected, or transmitted. Just like a fingerprint, each plant compound (chlorophyll, nutrients, stress markers) interacts with light in a unique way. Nutriscope™ analyzes these interactions to give you real-time crop health insights.Nutriscope™ operates using a three-step process that allows for real-time, non-invasive crop health analysis:
Emission of Light onto the Plant
The device emits light from a set of LEDs at specific UV, visible, and near-infrared (NIR) wavelengths. These wavelengths are carefully selected to target key biochemical properties of the plant, such as chlorophyll content, nutrient levels, and stress markers.
Reception of Refracted Light
As light interacts with plant tissue, certain wavelengths are absorbed, while others are reflected. Nutriscope™’s optical sensor precisely captures the intensity of reflected light across different wavelengths, allowing for detailed spectral analysis.
Calculation of the Absorption Curve
Nutriscope™ determines the absorption curve by analyzing the difference between emitted and received light. This curve serves as a unique spectral fingerprint, revealing the plant's chemical composition. By decoding these patterns, Nutriscope™ quantifies key plant health parameters, delivering actionable insights to optimize crop management.
Artificial Intelligence: Transforming Agricultural Data into Insights
Chlorophyll measurement is a clear example of how Nutriscope™ surpasses conventional methods
The way chlorophyll is measured highlights the key difference between traditional tools and Nutriscope™'s advanced approach. Conventional chlorophyll meters rely on just two wavelengths—one in the red and one in the near-infrared spectrum. They estimate chlorophyll levels using a simple ratio, which provides a basic but limited view of leaf greenness. While this method can indicate general trends, it does not capture the full complexity of plant physiology.
Nutriscope™ takes a different approach. Instead of relying on just two data points, it captures dozens of wavelengths across a broader spectrum. This provides a richer dataset, offering more information about the plant’s physiological state.
To analyze this data, Nutriscope™ uses machine learning, specifically deep learning, a type of AI that mimics how the human brain recognizes patterns. Instead of following a fixed formula, deep learning models process large amounts of spectral data, learning from real-world examples to improve their accuracy over time. This allows Nutriscope™ to identify complex relationships between wavelengths and chlorophyll levels, adapting to different plant species and growing conditions for a more reliable analysis.
By combining multi-wavelength spectroscopy with AI-driven analysis, Nutriscope™ provides a more detailed view of chlorophyll levels, helping farmers assess plant health and make informed decisions about nutrient management.
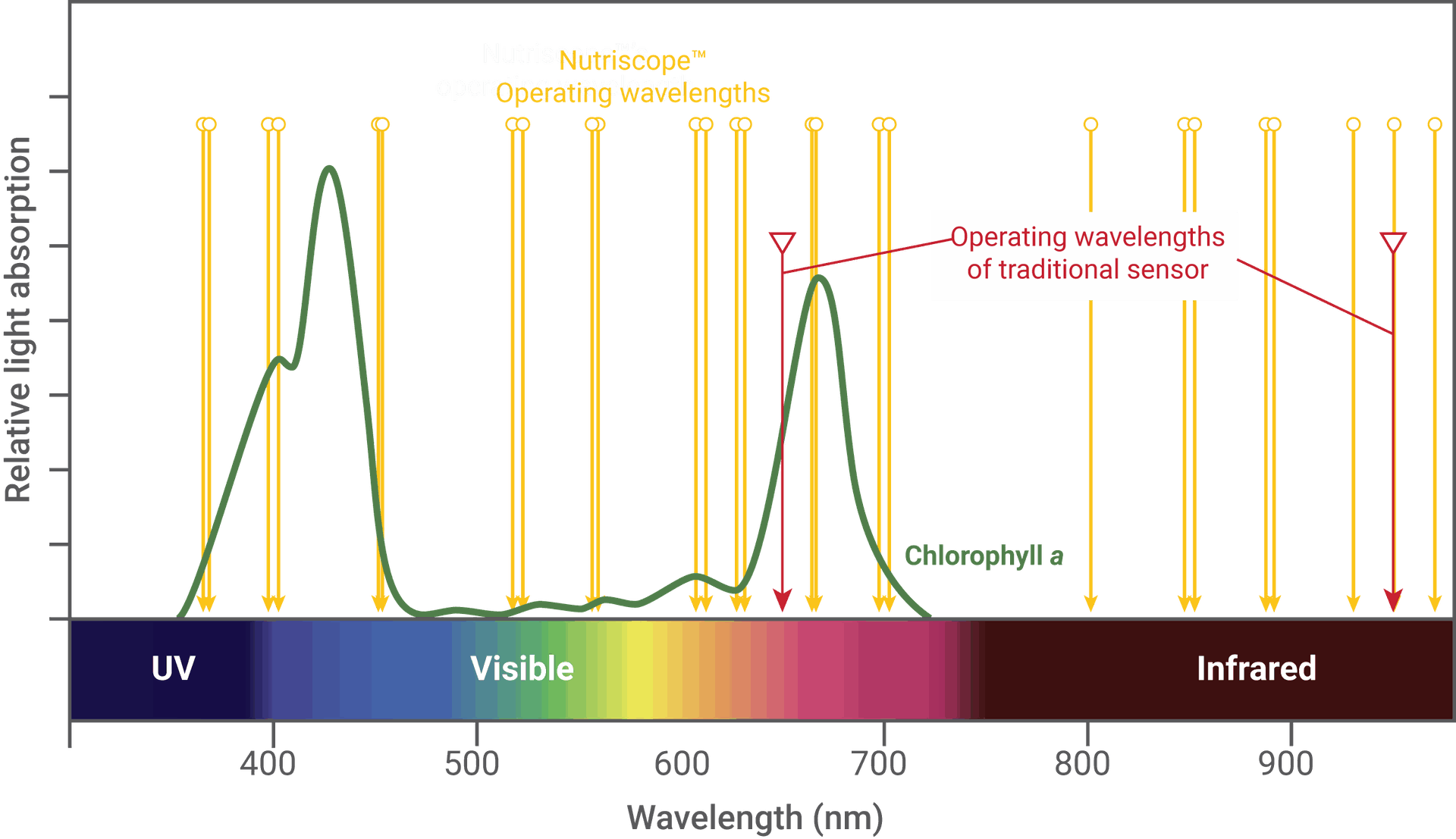
The Science Behind Nutriscope™'s Accuracy
Nutriscope™ applies machine learning (ML) to analyze plant and soil health, providing farmers with reliable insights. To ensure accuracy, our ML models go through a structured four-step process, combining field data with rigorous validation.
Data Collection
To train Nutriscope™ for a specific crop, we first collect absorption curves using Nutriscope™ on real plant samples. At the same time, we measure the same samples using reference instruments or lab analyses. This provides a high-quality dataset linking light absorption patterns to known nutrient levels, pH, or other parameters.
Data Cleaning & Pre-Processing
Before training the model, we carefully review the collected data. We remove obvious errors, such as misreadings or faulty measurements, and then format the data to make it suitable for ML processing. This ensures that only reliable, high-quality data is used.
Training the Machine Learning Model
We use 80% of the dataset (randomly selected) to train the ML model. During training, the model identifies patterns in the spectral data and learns to associate absorption curves with actual measurement values. Over time, it adjusts its internal parameters to improve accuracy.
Validation & Accuracy Testing
Once training is complete, we validate the model using the remaining 20% of the data, which was not used in training. This step ensures the model is making accurate predictions on new, unseen data. We compare the model’s predictions to the actual lab results and assess its accuracy before deploying it for real-world use.
Join the Future Innovations in Agricultural Technology
At Senseen, we believe collaboration is key to advancing agricultural innovation. While our Decision Support System (DSS) already integrates AI, solid agronomic science, and field expertise, we know there’s always more to learn and improve. That’s why we’re eager to create partnerships with researchers, agronomists, institutions, and industry leaders to develop new, crop-specific DSS solutions tailored to the diverse needs of farmers worldwide.
If you’re passionate about advancing smart agriculture and have ideas for collaboration, we’d love to hear from you! Whether you’re a researcher, agronomist, or part of an organization interested in co-developing the next generation of DSS tools, reach out to us today.